The edge of AI multi-strategy fund
- Hedge Capital
- Aug 31, 2023
- 5 min read
Updated: Sep 20, 2023
Why does it make sense to trade Bitcoin strategies? What are the benefits of multi-strategy funds for investors? What is the edge of a quantitative multi-manager model? How AI enhanced algorithms improve returns? Here’s a brief overview why such a fund is well positioned to produce sustainable risk-adjusted Bitcoin returns.
Why trading Bitcoin?
Because it’s the most rational choice from a risk/reward perspective for either type of crypto investor. First investor type aims to increase Bitcoin spot holdings as it constitutes an important part of his portfolio (for financial and/or ideological reasons). Aside from a new BTC spot purchase or non-BTC investments, such an investor may increase his BTC capital in risk-adjusted manner only via BTC denominated strategy. Hence the reason to create Alpha BTC, an “all-weather” multi-strategy portfolio trading BTC inverse derivatives (i.e. denominated in BTC).
Second investor type has the ultimate financial reason to generate outsized dollar profits compared to traditional markets in return for accepting higher capital volatility. Rational and risk averse investor does so first by investing in spot Bitcoin (the least risky crypto asset creating base of most crypto portfolios). Those seeking even higher returns may venture into altcoins and trading derivatives, both being essentially a leverage play on Bitcoin itself. While trading and especially derivatives is not attainable for most investors, investing in altcoins seems to be, but offers no diversification, low risk-adjusted returns and unnecessary centralization risks. See in numbers in our Benchmarks analysis why most fundamental funds are just a riskier proxy to ETH itself. Assuming rationality, the investor should therefore either hold spot ETH and accept its risks in return. Or invest part of spot BTC holdings into a strategy with significantly higher risk-adjusted returns than offered by ETH. Hence again the reason to create Alpha BTC, a portfolio of diversified derivative strategies with BTC risk-adjusted returns 5x higher than ETH.
Why multi-strategy?
Trading derivatives is inaccessible to smaller HNWI investors lacking expertise, while institutional investors such as funds, miners or exchanges seek diversified products with consistent returns and low capital volatility. Those are typically not found in single quant strategies that often only extend the same algorithm to several altcoins, increasing counterparty and asset risk without improving risk-adjusted returns and diversification. Investors interested in increasing yield from their BTC holdings, via a diversified portfolio of quantitative strategies, have today few options to choose from.
Bitcoin market character is evolving along with its transition to mainstream finance, through the introduction of derivative and investment products and its increasing adherence to traditional markets. This mix of organic evolution and inorganic sudden changes increases unpredictability on the back of ever increasing efficiency of Bitcoin spot and derivative markets. To consistently capture alpha (i.e. returns above BTC benchmark) in such an evolving environment over a longer period of time, the investment strategy requires uncorrelated diversification.
For the diversified portfolio to retain its edge, it should span across products (options, swaps), timeframes, strategy types and quant approaches. Though not a guarantee and adding to the complexity of managing such a portfolio, AI enhanced algorithms may also increase and sustain the ability to exploit market inefficiencies. As such diversification is hard to achieve within the means of a single quant team, Hedge Capital adopted a more flexible and sustainable approach to build its multi-strategy portfolios using selected third-party algorithms, thus adding quant team capacity and diversity into the portfolio diversification as well.
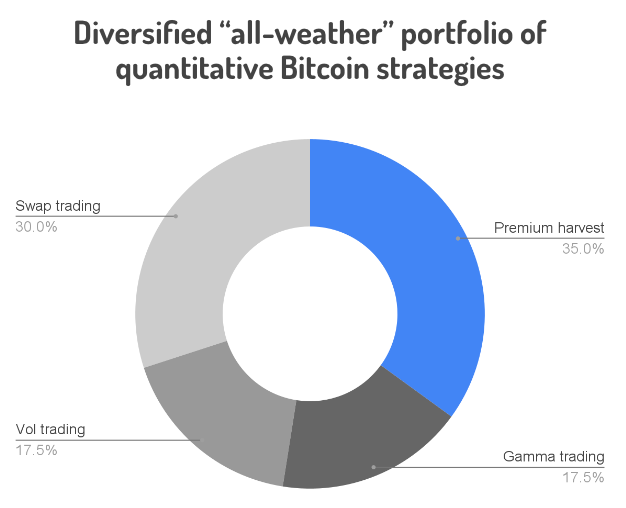
In short, Alpha BTC multi-strategy enables to exploit portfolio theory, i.e. achieve better risk-adjusted returns via diversification and make it accessible to investors unable to access or manage an advanced portfolio of options and swaps derivatives. In particular, such portfolio provides investors with:
Diversification - Inexpensive and efficient way to diversify investments via negatively correlated quantitative ML strategies. Negatively correlated that hedge each other to perform in various market contexts, to reach ideal return profile resulting in high risk-adjusted returns (Sharpe ratio).
Quant expertise - quant finance domain expertise unavailable to HNWIs, miners or discretionary hedge funds in order to perform well the core function - quant strategy and understanding and selection.
Access thresholds - high capital requirements made accessible to smaller investors optimizing their risk/reward.
No netting risk - investors are charged performance fees on fund level, that is, on netted result of all sub-strategies with the fund bearing the netting risk. This mitigates investors’ risk of paying performance fees on individual strategies despite the aggregate portfolio position being in loss.
Portfolio management - ongoing performance and risk management, ongoing portfolio development and monitoring.
Counterparty risk management - opaque inefficient industry requires ongoing quantitative, technological and legal / compliance due diligence.
To successfully manage such a portfolio, the Hedge Capital team leverages decades of experience in quantitative finance, quant trading, fintech development, asset management and legal practice. For the initial Alpha BTC portfolio, the team reviewed ~700 crypto hedge funds, shortlisted and reviewed in detail about 30 investment managers and their quantitative strategies. The fund is actively reviewing existing algorithms and teams on the market in order to further increase diversification of the portfolios.
Why quantitative multi-managers?
Answering the quantitative vs fundamental question is fairly straightforward. Statistically, about 95% of fundamental discretionary funds (those that manually pick assets or trade) lose over the long term via subjective emotional decisions, incompetence and bad risk management. They trade without a sustainable statistical edge at best as a more volatile BTC or ETH proxies, see again Benchmarks.
Quantitative funds, on the other hand, face a brain power capacity dilemma. For a quant strategy to sustain its edge, the fund needs continuous work of several quants. The more quant strategies the fund runs, the more quants it needs. As a result, small quant funds either run one or few strategies at best or face a dilemma to grow the quant team substantially to achieve diversification.
Small quant teams - Limited resources focused on single strategies, limited portfolio and risk diversification.
Several quant teams - Tens of independent teams in multi-manager model or handful of teams within a large proprietary trading firm
Crowdsourcing quants - Numer.ai trading hundreds of stocks with a meta-aggregate of thousands of quant models
Hedge Capital multi-manager model offer diversification also on quant team level, flexibility to choose the best algorithms from the best teams without being limited on brain power capacity. To manage such a model, Hedge Capital leverages its own internal ability to build and trade quantitative algorithms in options and swaps. It enables the team to “ask the right questions”, look under the hood and handle the complexity of a quant counterparty due diligence.
Why AI enhanced algorithms? In general, AI enhanced algorithms may, if implemented and developed well, improve quantitative modeling by providing deeper insights, increase trading signal efficiency and help to retain trading edge by continuously adapting to market changes. While humans bring domain knowledge and strategic oversight, AI provides the ability to process vast amounts of data and identify patterns that humans might miss.
Specifically, Alpha BTC and Alpha USD portfolios include third-party quantitative algorithms that are either enhanced or fully based on Machine Learning (ML), a term often used interchangeably with AI. In particular, option strategies use ML to scan options implied volatility surfaces to pick the most inefficient pricing (enhances quant model) while the swaps long/short strategy uses ML for price predictions (powers quant model).
On the flip side, AI quant algorithms, especially those using deep learning models, may lack explainability of their decisions, are prone to overfitting and depend on quality data. An understanding of those risks and limitations is crucial. The challenge is to find ML based quantitative strategies and quant teams that have been able over an extended period of time to develop and trade in various market contexts profitable strategies while mitigating the cons above. To assess that, the Hedge Capital team again leverages its diverse backgrounds, especially in mathematics and quantitative finance.